Building AI Products: Learnings from a Panel with Advancing Women in Product
Sift is passionate about education, collaboration, and empowering innovation. Last month, Sift partnered with Advancing Women in Product to host an event at our new office in Downtown Seattle. This was also the first event for AWIP’s newly-founded Seattle branch, so energy was high as the community embraced this awesome opportunity. 50+ attendees gathered at Sift’s office for a panel of product managers from Sift, UiPath, Amazon, and Microsoft who shared their experiences on Applied AI for Products.
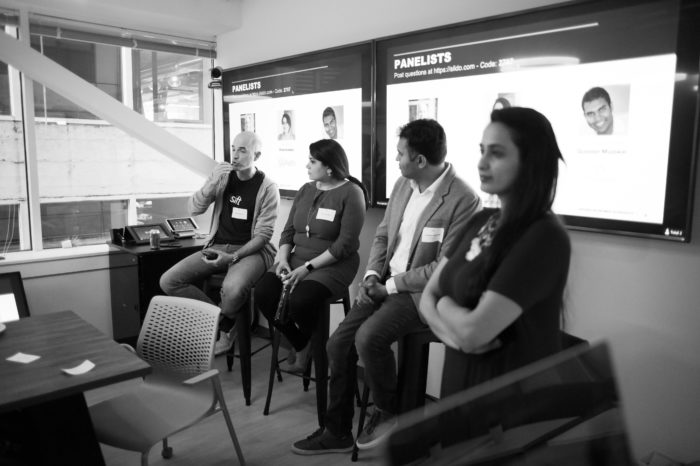
What did we learn?
Artificial Intelligence (AI) is not magic. Rather, it’s all about automation; getting computers to perform repeatable, simple tasks that humans can do easily. Automation provides the speed and scale required to support applications like real-time fraud protection (Sift), customer support (UiPath), and finding a safe and cheap gas station without taking your eyes off the road (Amazon Alexa).
AI products have similar elements to other products: they need to solve real customer problems, be easy to use (UX is king!), and provide evident value to customers. But the dependency on data sets these products apart. Getting computers to correctly perform these tasks requires a lot of data – sometimes billions of examples – from millions or more users. Additionally, you typically don’t hit the performance you were hoping for on the first try; good models require many iterations to train (also known as “experiments”), as data needs to be cleaned and parameters tuned. Since most of your experiments will fail, a good AI team has a way of scaling this process so it’s cheap(er) and easy(er) to experiment again and again until you achieve consistent, desired performance.
Getting to work
If you’re a PM or engineer looking to get into AI, panelists had a few recommendations:
- Start with the customers (or the problem) rather than with the tools: Find problem that is meaningful to them and you and immerse yourself in that respective domain.
- Learn more, be curious, apply your knowledge: Read an intro book on AI or ML, or take an online class to understand the possibilities. Then find the opportunity to work on a solution by joining an existing team or starting your own.
- Hire smart: If you’re building AI teams, understand your real need and go for balance, not hype. “Data scientist” and “ML engineer” are key roles in AI R&D, but there are additional roles that are crucial for getting an AI product out: backend engineers, UX designers, PMs and more.
- Think about the now: Consider the mix of skills at any given point in time. Are you a mature organization with stable experimentation platform? You might benefit from hiring more data scientists to extract knowledge from the data. Or are you a team who needs to build this platform first? Perhaps you should primarily hire engineers who can write backend production code.
What’s next? We’re grateful to AWIP for their partnership and glad to have had so many attendees. Looking forward to hosting more events at our Seattle office, so stay tuned.
Interested in learning more about opportunities at Sift?
Visit www.sift.com/careers